Machine to build the machine: Automation key to Gigafactory success
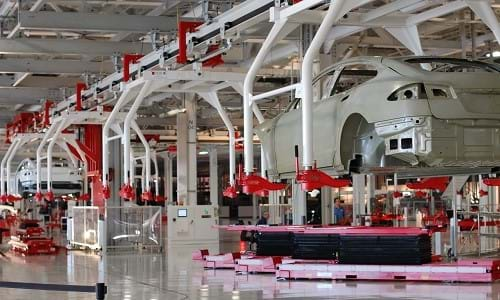
Machines with the ability to learn might seem like something from a far-off sci-fi future, however many industries are affected by, and can benefit from, machine learning and 2017 will be the first year we’ll actually start to see the real world impacts of machine learning technologies. Automation is integral to manufacturing, and machine learning has the potential to take things to the next level. Manufacturers will need to keep up, and it is driving their reliance on data centres like never before.
Manufacturers are even now able to collect vast amounts of data every hour, minute and second from sensors attached to their production lines. This data can be used to enhance operations, handle equipment issues or potential problems with them, and meet various goals that can help increase capacity and drive better margins. But the problem has always been analysing the data and turning it into actionable information in a timely and cost efficient way.
This is where machine learning comes in. It is a subfield of artificial intelligence (AI) that attempts to give computers the ability to learn from experience, to be pattern-recognition machines. That makes it sound pretty simple, but it gives manufacturers the power to optimise their operations by learning patterns via observation of the production line and associated data streams. Much faster than any human, machine learning can analyse these immense amounts of data in real-time and turn it into actionable improvements that can speed production cycles and lower costs by avoiding errors and mitigating anomalies.
All manufacturers have the potential to integrate machine learning into their operations, allowing them to gain predictive insights into production and thus become more competitive. For an industry that hinges on efficiency and optimisation, this is a big deal. Increased production yields through optimisation are already making a difference for aerospace and defence, industrial and high-tech manufacturers today. Manufacturers are able to use more of their production capacity, and using machine learning to help optimise the best possible combination of machines, staff, and suppliers.
That all sounds exciting, but the explosion of machine learning has led to the need for specialised computing infrastructure that can handle the workload. These computers are similar to the high-performance computing (HPC) systems used by scientists, and require power, cloud storage and cooling capacity from data centres. For example, Graphics Processing Units (GPU) are one of the things that make HPC systems tick. They are essentially virtual neurons, but unlike the human brain, they use extremely large amounts of electricity.
As more and more manufacturers require the use of high tier data centres to fuel their machine learning data needs, having connectivity to reliable power will be integral for data centres in the future. Being able to provide reliable power infrastructure will dramatically improve performance and allow manufacturers to process more data. The good news here is that data centres can also make use of machine learning to understand where improvements can be made to their own systems. Google have used it for their own data centres to make power savings that, while small on paper, will have a huge cost saving affect when applied across their tens of thousands of servers.
Manufacturers can collect vast amounts of data, but more and more they will need to rely on data centres with smart, efficient infrastructure. The technology is still young, so it is not clear what will be required of data centres in the future, but what is clear is that data centres will need to be able to handle the 24/7 needs of manufacturing clients across the globe as machine learning for automation becomes the norm.